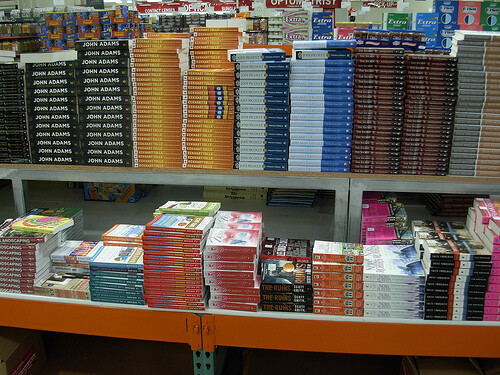
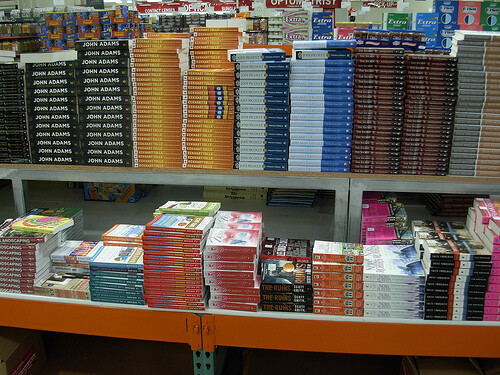
Big data, with all of its vastness and mystery – and confusion – is actually a remarkably straightforward concept: store everything for later analysis. Because once it’s gone, you can’t get it back. The end.
Okay, so there’s more to the big data story. And while the term big data is both overexposed and under-defined, defining it doesn’t really help solve business problems. (And we won’t even get into Big Data vs. big data, because who really cares except for data practitioners.)
But recounting a business success story that substantiates the notion that you should store every last iota of data – because you don’t know every pattern [read: opportunity] that might be found or explored – should shed some bright light on the awesomeness that is big data.
The Big Challenge
An online bookseller didn’t know its profit margin on any individual sale, and therefore did not know where to set its price floor on the marketplace to stay competitive. How could they:
- Accurately predict a total cost per book?
- Determine a price floor?
- Price competitively against that floor on the marketplace?
- Gain a competitive edge?
- Sell more books?
- Maximize profits?
The Big Problem
Profits can only be realized after reconciliation. But reconciling costs, from cradle to grave, just wasn’t feasible. Too much data and too many variables in two separate ecosystems (supply chain and marketplace) made for too much guesswork in projecting costs and setting prices, and left the bookseller at the mercy of the marketplace and its competitors. Some of the data points to be reconciled, included:
Inventory:
How much did each book cost? How would the exchange rate complicate everything? How many were actually purchased, and also delivered? Is there excess inventory? What about returns? Do you resell them or do the returns become dead inventory?
Delivery:
What was the real cost of transit? How were changing gas prices accounted for? Was there a gas surcharge? Did the bookseller pay a bulk rate or was the volume too low to qualify? How would quality and speed of delivery affect the feedback rating to avoid getting kicked off the exchange? Did the load move through a transient storage facility? Was a different shipping rate applied from point B to point C? What about the rates for different delivery vehicles – planes, trains, boats and trucks?
The Big Opportunity
The opportunity was this: Any time you can quantify a cost or a risk, you can use that data to feed back into your algorithm for determining the floor of your product. Then you can price more aggressively and ultimately take business away from a competitor.
Since they didn’t know ahead of time which data would help quantify that cost or risk, the bookseller stored everything. Because once it’s gone, it’s gone. This big data approach represents a paradigm shift from a traditional data approach, whereby you first figure out what you need and store only that. (To reiterate: we’re not concerned here whether it’s defined as Big Data or big data.)
*The bonus of a big data approach: it’s more fun, because everyone gets to add their two cents about what’s going to be valuable downstream, for only a nominal cost.
After exhaustive data cleansing and analysis, and then feeding that information back into the pricing algorithm, the bookseller now had created a homeostatic supply-chain and logistics cost-predicting machine!
The Big Payoff
Like the supply chain ecosystem, the marketplace is filled with data to be collected, manipulated, and analyzed – with nearly unlimited capacity to create competitive edge. With the intelligence gathered from the awesomeness of the cost-predicting machine, the bookseller could now focus on leveraging that cost-predictability into book sales.
An online bookselling exchange compares very neatly to any active trading market, e.g. stocks, commodities, etc. You set your prices and then set up the dogfight rules! In simplest terms, the bookseller had to be faster and smarter than its competitors so it could sell more books.
Now that the bookseller could set the price floor on any single book (thank you, supply chain data machine extraordinaire) the dam was burst, and the opportunity on the marketplace exploded. The accuracy of being able to predict actualized costs allowed for aggressive pricing without putting the whole business at risk.
The bookseller’s high-speed transactional system collected price change data on 5 million SKUs of books daily (over 150 million prices) and could instantly respond to penny changes in the price of any one book.
Because the bookseller could predict and respond to price changes so much more quickly than competitors, the bookseller propelled itself into a position where its books were the most likely to show up as the lowest price during 90% of the day.
So at the end of the day (literally) they’d win more transactions because they had the lowest prices for the longest period of time.
Oh, and one last piece of data triumph – this bookseller vaulted from obscurity to a top 10 online seller of books in a little over 18 months.
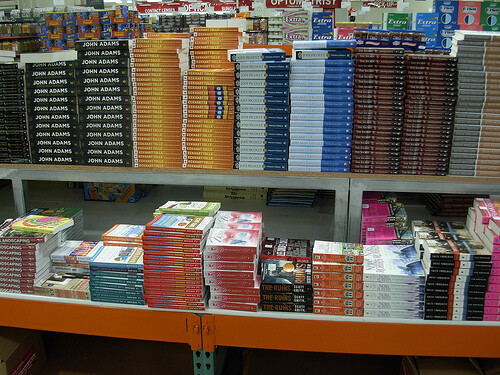
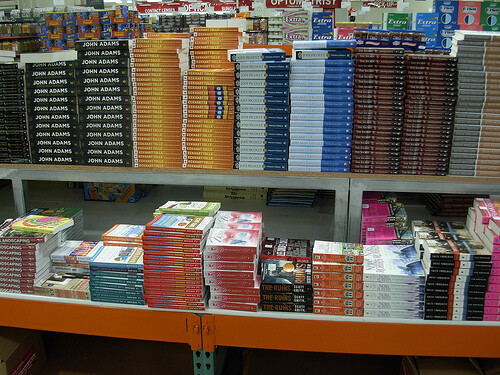
- Accurately predict a total cost per book?
- Determine a price floor?
- Price competitively against that floor on the marketplace?
- Gain a competitive edge?
- Sell more books?
- Maximize profits?